Confirmation Bias: Check and Balance Processes
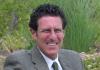
COVID19 has exposed confirmation biases among some expert scientists. Confirmation bias occurs when people actively search for and favor information or evidence that confirms their preconceptions or hypotheses while ignoring or slighting adverse or mitigating evidence. It is a type of cognitive bias (pattern of deviation in judgment that occurs in particular situations - leading to perceptual distortion, inaccurate judgment, or illogical interpretation) and represents an error of inductive inference toward confirmation of pre-existing world-views (or hypotheses).
Dr. John Ioannidis' 2005 paper "Why Most Published Research Findings Are False" provides strong evidence of confirmation bias among professional scientists. Ioannidis analyzed 49 of the most highly regarded research findings in medicine over the previous 13 years. He compared the "45 studies that claimed to have uncovered effective interventions with data from subsequent studies with larger sample sizes: 7 (16%) of the studies were contradicted, 7 (16%) the effects were smaller than in the initial study and 31 (68%) of the studies remained either unchallenged or the findings could not be replicated."
In applied data science, providing clients/employers with flawed data science results may lead to bad business and policy decisions. Cherry-picking data in collecting and focusing on some evidence to the exclusion of other evidence leads to an illusion of reality and potential disaster.
Professional data scientists create check and balance processes to guard against confirmation bias. This means actively seeking both confirmatory and contradictory evidence and using scientific methods to weigh the evidence fairly.