Models vs. Experiments
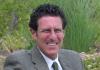
At the Data Science Association we see many organizations spending a majority of data science team human capital and time building models. While models can be useful in seeking to understand complex phenomena, all models are flawed and present an illusion of reality. This is especially true in high causal density environments (e.g., human behavior, finance, climate, health, public policy).
I respectfully suggest that organizations would produce more valuable, actionable insights by spending less time building models and more time conducting experiments. The recent datafication of everything plus cheap compute power and storage makes conducting many low-risk, cost-effective experiments a reality for organizations of all sizes.
The implicit assumption in building and relying on models is that if you understand complex relationships and find patterns and correlations, you can make better decisions, forecast events and manage risk. As we all know, the real world is not that simple and causes are usually obscure. Critical information is often unknown or unknowable and causes can be concealed or misrepresented. Moreover, key assumptions embedded in models are often wrong. In high causal density environments, finding true causality is difficult and sometimes impossible.
The solution is to use the recent abundance of cheap data and compute power to design and execute many low-risk experiments versus spending time building models. The trick is to integrate nonexperimental (models) and experimental methods.
Decision makers can improve decision making and prediction methods by conducting more experiments. Yet limits to the use of experiments are established by the need for leadership, strategy and long-term vision. Business and pubic policy leaders need to support and adequately fund experimentation by the data science and business analytics teams. Data scientists need to master the science of designing and executing experiments and spend more time and brainpower conducting low-risk experiments and less time building models.
True randomized experiments are most reliable: the randomized experiment is the scientific gold standard of certainty of predictive accuracy in policy and business. If a program is practically testable and the experiment is cost-justified (i.e., expected value of information worth cost of test), experimentation is the best method of evaluation and prediction.
For smart, high-value data science teams, designing and executing many low-risk experiments - in addition to using models - is often the best strategy.